Towards Rigorous Frameworks for Scientific Machine Learning: Theory and Applications for Multi-Scale Chaotic Dynamical Systems
Events | Mechanical Engineering
Towards Rigorous Frameworks for Scientific Machine Learning: Theory and Applications for Multi-Scale Chaotic Dynamical Systems
May 1, 2023 8:30 AM
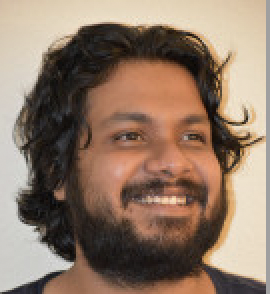
Speaker
Dr. Ashesh Chattopadhyay
Location
ESB 1001
Type
Seminar
Despite consistent improvements in modern computing infrastructure, predicting the evolution of the states of multi-scale high-dimensional chaotic dynamical systems, such as the Earth’s weather, climate, large-scale engineering systems, and their extremes, such as extreme temperature, wildfire, flooding, etc. remains a grand challenge in natural science and engineering. In recent times, with an influx in high-quality observations and high-fidelity simulations, data-driven methods, primarily fueled by the unprecedented success in deep learning, hold significant hope for modeling and predicting high-dimensional multi-scale PDEs up to the smallest scales. However, off-the-shelf deep learning algorithms that have received significant success in the computer vision and the natural language communities cannot directly be applied to scientific problems. To that end, this talk would primarily revolve around building principled scientific machine learning frameworks at scale, wherein scientific priors/structure, inspired from physics and numerical methods, in the form of inductive bias, novel regularization, etc., can be baked into the deep learning architectures. We would explore different failure modes in the form of instabilities and their mitigation strategies in scientific machine learning models of PDEs, applications to data assimilation in high-dimensional chaotic systems, and finally build an “explainability” framework for out-of-distribution generalization of deep hybrid (deep learning and numerical) models of multi-scale PDEs, grounded in physical and deep learning theory.