ME Seminar on "Coarse-graining the dynamics of (and on) complex networks "
Events | Mechanical Engineering
ME Seminar on "Coarse-graining the dynamics of (and on) complex networks "
April 28, 2014 4:00 AM
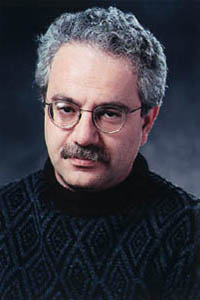
Speaker
Yannis Kevrekidis, Princeton University
Location
**ESB 2001**
Type
Seminar
Abstract: Complex, large scale networks often dynamically evolve in time. One can discriminate several different forms for such an evolution: (a) dynamics ON networks, when the connectivity of the network is fixed, but properties of the nodes evolve (e.g. concentrations in a complex biochemical reaction network); (b) dynamics OF networks, where the connectivity of the network itself is evolving – edges either form or disappear in time; finally (c) both properties of the nodes and existence/weights of edges evolve, giving us dynamics "of and on" networks, sometimes termed, adaptive network dynamics.
I will begin by describing an approach to coarse-graining the dynamics of large networks whose connectivity changes dynamically. The approach is formulated within our equation-free framework, given a full, detailed model of the network evolution dynamics. We assume that we know what the crucial macroscopic network features are, the "dependent variables" in terms of which we can write macroscopic evolution equations (e.g. the network degree distribution). We then perform short bursts of detailed network evolution simulations from which we estimate the right-hand-sides (and the actions of the Jacobians) of the unavailable closed "macro" evolution equations on the fly. The approach involves repeated use of lifting and restriction operators that translate between actual network realizations and their (appropriately chosen) coarse observables. I will show how to accelerate temporal simulations (through coarse projective integration), and to implement coarse grained fixed point algorithms (through matrix-free Newton-Krylov GMRES). The scope and applicability of the approach will be discussed - including an interesting "technology transfer" between heterogeneous network dynamics and uncertainty quantification algorithms.
I will then discuss the selection of "the right macroscopic" variables based on data-mining mining tools, in particular manifold-learning techniques like Diffusion Maps. We extend these data mining approaches to cases in which every data points in a time series is a "snapshot" of an evolving graph. We are thus able to detect intrinsic low-dimensionality in ensembles of graphs, and detect the appropriate coarse variables. One of the main challenges in mining graph evolution data is the definition of a suitable pairwise similarity metric in the space of graphs. We explore two practical solutions for this problem: one based on finding subgraph densities, and one using spectral graph information. We demonstrate the data-mining process by detecting and parametrizing low-dimensional families of graphs arising from graph construction algorithms as well as from dynamic graph evolution laws.
Bio: Dr. Ioannis (Yannis) Kevrekidis is the Pomeroy and Betty Perry Smith Professor of Engineering at Princeton University. His research focuses on scientific computation for complex/multiscale systems modeling, process dynamics, computer modeling, and applied mathematics, spatiotemporal pattern formation, and nonlinear system identification and control. Professor Kevrekidis has published over 300 journal articles in a broad range of top journals in Applied Mathematics, Chemical Engineering, Fluids, and Physics. Among his numerous distinctions, he has received a Microsoft Visitorship from the Isaac Newton Institute, the Gutzwiller Fellowship from Max Plank Institute PKS, the AICHE Wilhelm Award, the Guggenheim Fellowship, the SIAM Crawford Prize, and Alexander von Humboldt Foundation Senior Research Award, and is a Fellow of SIAM.
Host: Francesco Bullo